From snow to plastic road markers, self-driving vehicles still face major tests
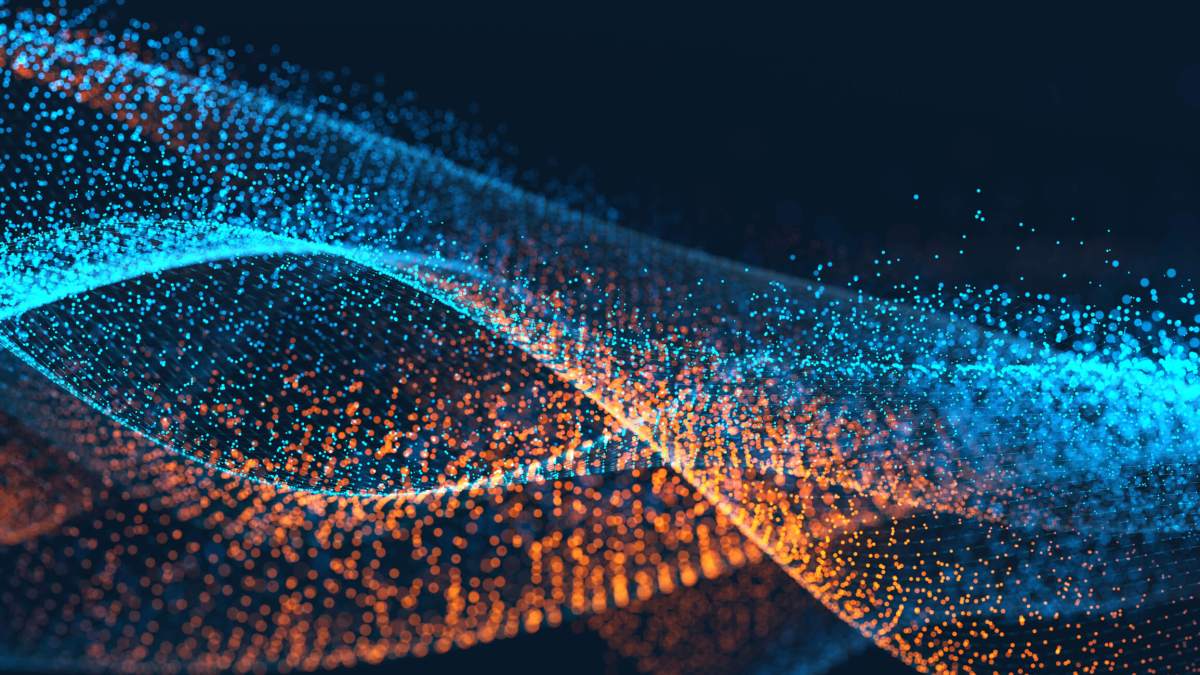
In the mind of the public, self-driving vehicles are coming soon to America’s roadways. Manufacturers, researchers and policy-makers, however, have a few speed bumps ahead before the cars are driving around en masse.
“From a technological perspective, we’re not there yet,” Bryan Reimer, the director of MIT’s AgeLab and associate director of MIT’s New England University Transportation Center. “How capable are those vehicles of providing ubiquitous autonomous driving? I think that’s an open question.”
Reimer said he does expect to see self-driving vehicles firmly in the marketplace at some point, and manufacturers certainly are locked in a race to advance autonomous vehicle technology through the use of advanced radar and imaging technology, deep-learning machines and the introduction of semi-autonomous features.
In September, Uber rolled out a fleet of its self-driving taxis in Pittsburgh with each car requiring two Uber employees in the front seats at all times. In June, General Motors finished its second fleet of near-Level 4 autonomous vehicles — vehicles that can operate nearly on their own, but require a human behind the wheel in case of emergency — and has plans to deploy a number of vehicles to Arizona, which has become a hotbed of autonomous vehicle testing. The relaxed regulations there and the flat, predictable weather of the desert provide ideal conditions for companies to get mileage on their test cars.
When will it all be ready for everyday use, though? It’s a question of standards, Reimer said.
“We hold robots to a higher body,” Reimer said.
Everyday situations that humans navigate from behind the wheel — a sudden change in the weather, a complex intersection or a split-second swerve to avoid an obstruction — are overcome within a certain margin of error.
But the expectation is that self-driving cars must be perfected beyond humanity’s margin of error, because in our eyes, “Robots should be perfect,” Reimer said.
The scope of research into solutions to these problems is staggering. Something as simple as a rain shower can be big trouble for the technology that helps a self-driving vehicle navigate.
Eyes on the skies (and the ground)
One of the primary concerns for researchers and manufacturers is developing secure and road-tested protocol for inclement weather, which can be a struggle for a vehicle that relies on advanced technology to sense the environment around it.
It’s bad enough for humans already. The Federal Highway Administration (FHWA) estimates that of more 5 million different crashes a year, 22 percent are directly related to inclement weather, resulting in roughly 6,000 deaths per year. This data was collected from 2005 to 2014 — perhaps the last decade the results will not include significant numbers of semi- or fully-autonomous vehicles.
Santhosh Tamilarasan, a graduate student and researcher at Ohio State University’s Center for Automotive Research, told StateScoop researchers are making progress.
“If there’s going to be a bunch of snow that falls on the camera, then the car will lose its vision,” Tamilarasan said. “The vehicle will use other sensors, like Radar and Lidar, to actually get information about the road and to use the landmarks to predict where the car is located to navigate. We call this sensor fusion — we try to fuse the information from different sensors, so that even if one of the sensors fails for any reason … we can collaborate the information from the other sensors and then try to use that to verify where we are.”
Lidar is the boxy sensor on top of many self-driving vehicles in testing phases today. It offers a constant 360-degree view of the area surrounding the car and emits millions of beams of light per second, all invisible to the human eye. The Lidar sensor uses the refractions of these beams to construct a true 3-D image of the area surrounding the car, offering a hugely valuable navigation source.
Without use of their cameras, self-driving vehicles are forced to rely on alternative sensors and landmarks to position themselves on the road — technological processes that haven’t yet been heavily tested on public roads.
“What TomTom and other mapping agents are trying to see is, ‘Can I actually have Lidar on top of the car, go on the road and capture all the Lidar images, and then since I know where I’m traveling as part of the mapping exercise, use that Lidar to map the information,” Tamilarasan said. “That way, even if my GPS fails, I can use it to actually accurately locate where I am.”
The machine learning way
Weather isn’t the only factor on roads liable to change at a moment’s notice, however. While self-driving vehicles can obey traffic laws in a closed-system trial without fault, any road with human drivers will be a space prone to unpredictable situations that require abstract solutions. Virtually every intersection will bring about a new situation, and as Tamilarasan explains, there are basically two different methods of teaching self-driving vehicles how to adjust.
“One is the robotic way of doing it, and the other is the artificial intelligence way of doing it, or the deep-learning, machine-learning way. The robotics way of doing it is the more conventional way — you get all the information, you have a set of orders, and once you have all the information, you have a flow chart of steps that can be taken,” Tamilasaran said.
Using this system, Tamilarasan said, companies can actually go back and trace where the car made an error by following the functional flowchart of decisions based on the data that the car had at the time.
“The other way of doing it is artificial intelligence or machine learning,” Tamilarasan said. “You take all the information from all the sensors, and you have a black box, which is basically the deep-learning network.”
Self-driving vehicles that operate on such a system are trained by watching videos from a dash cam and the car will respond to the actions shown in the video.
“Once you show all the driving videos to the deep-learning network, basically, the car is able to learn from it,” Tamilarasan said. “It will actually learn from it and when you put it in a real scenario, it will try to mimic the actions in the video.”
Stop signs, construction zones, low-speed areas and many other common driving situations figure into the learning process for vehicles trained via machine learning.
“The only caveat is that deep-learning operated cars do not have a strict pattern of decision making, limiting the potential for investigators to understand what went wrong in a crash,” Tamilarasan said.
Where the dots meet the road
The roads that self-driving vehicles will share with manually driven cars could also pose problems with the current technology available to manufacturers. While the development of self-driving technology is largely spurred and dictated by the private sector, America’s public road system will likely be the constant in the equation of the two.
If vehicle manufacturers want large-scale efforts to radically change the nation’s driving surfaces — even if only in the name of safety — it won’t be easy.
“Roads are difficult,” Reimer said. “Roads require enormous infrastructure spending, and it’s unlikely to occur. Self-driving vehicles are probably going to have to work within a road infrastructure that looks like the one we have today.”
California’s Director of Transportation, Malcolm Dougherty, has supported widening the lines between lanes and moving away from California’s system of Botts Dots — the bumps that provide an auditory cue to drivers who drift outside their lanes. While self-driving vehicles can spot details like these, both changes would theoretically improve the ability of the vehicles to visualize roadways.
The defining question for self-driving vehicle development, however, doesn’t revolve around a single technical function, and won’t have an answer for at least a few years, Reimer said.
“The societal question of ‘how safe is safe enough’ is the toughest thing we have to solve,” Reimer told StateScoop.
The famous “Trolley problem” is often referenced as an ethical dilemma in which self-driving vehicles will find no agreeable solution. The problem forces a vehicle to place different values on various individuals lives, inevitably making a decision of which individuals to crash into and which to save. As philosophers continue to debate the preferred outcome for such a scenario, the prospect of placing that decision into a robot’s hands is uncomfortable for many.
As Reimer’s team at MIT found in their study, many more people are comfortable with various levels of automated assistance — like lane-merging and brake assistance technology — than full automation. Despite the hesitation, Reimer told StateScoop that those nervous about sharing the road with a fully automated vehicle don’t need to worry until the technology catches up to the constraints. He also emphasized that it’s not going to happen overnight, likening development of the technology to an “evolution, not a revolution.”
“That ethical dilemma is a future problem, but it’s out there,” Reimer said. “Until we have the computational logic and the capabilities to make decisions at that level, it’s a moot issue. I feel confident we will get there at some point in the distance, but at this point, the decision logic can’t tell if it’s an older adult versus a younger adult — we’re just trying to see if it can detect the pedestrian.”