In first meeting, NYC algorithm task force talks racial biases, ‘dirty data’
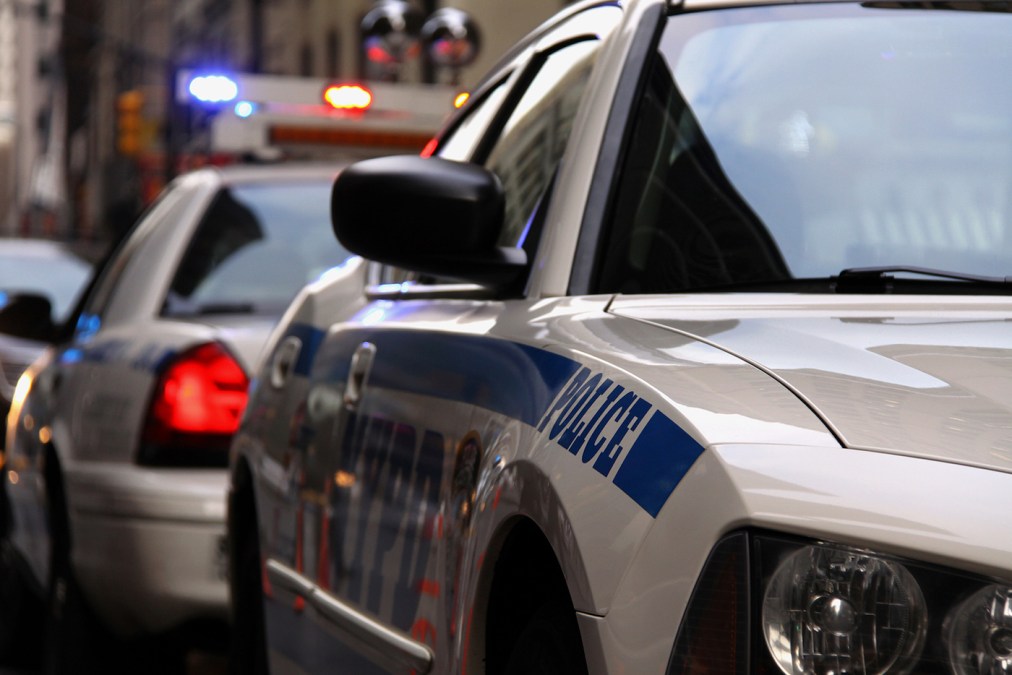
A task force charged by New York Mayor Bill de Blasio last year to evaluate the city’s use of algorithms held its first meeting Tuesday night. In a two-hour meeting in lower Manhattan, the city’s Automated Decision Systems Task Force heard from a handful of expert witnesses who described how blind trust in computer programs meant to make public officials’ jobs easier can create unfair and sometimes unconstitutional policies, especially pertaining to law enforcement.
“The amount of data we have grows exponentially, but our capacity as humans to fully comprehend it does not grow,” Andrew Nicklin, a “futurist-at-large” at the Johns Hopkins University Centers for Civic Impact, told the task force. “So we need tools to understand it.”
Nicklin’s think tank, which was rebranded this week, contributed to a “toolkit” last year designed to help cities adjust the algorithms they use by putting the underlying data into better context, instead of a “black box” approach in which decisions are made without any closer evaluation.
De Blasio created the task force last May after the New York City Council passed legislation mandating a review of the city’s automated decision systems. The law’s author, Councilman James Vacca, said he was inspired in part by a 2016 ProPublica report that found that algorithms used by law-enforcement in New York and other cities routinely assign higher risks of recidivism to black people than to whites.
In his testimony Tuesday evening, Nicklin cited “risk scores,” which are designed to inform judges before sentencing criminals of the likelihood that the defendant will be a repeat offender. The metrics behind such scores often assign higher risks to youths, black and Hispanic individuals and people from low-income neighborhoods, frequently leading to harsher sentences. But Nicklin suggested judges tend not to override those algorithmic decisions because they don’t understand the process.
“We know in some cases that judges often rely on risk scoring that they see without necessarily understanding the mechanisms that are coming up with those risk scores,” he said.
Nicklin conceded automation is necessary to help governments sift through ever-growing mountains of data, but said there should still be openings for “human intervention” to make corrections, or to simply help officials understand the effects of the decisions being made.
Meanwhile, Janai Nelson, the associate director of the National Association for Advancement of Colored People’s Legal Defense Fund, brought the task force several recommendations to reform the use of automated decision systems by city agencies, particularly the New York Police Department. In particular, Nelson urged the panel to recommend that the NYPD not use algorithms fed by data collected through the the department’s long-standing “stop-and-frisk” tactic, which a federal judge ruled in 2013 violated the rights of the city’s minority residents.
While the policy has been largely abandoned since the ruling — the number of stops fell 98 percent between 2011 and 2017 — the NYPD still conducted more than 4.4 million stops between 2004 and 2012, with more than half on African-Americans but only 10 percent on whites, and with nearly 90 percent of stops not resulting in any actual enforcement action. The datasets from those stops still exist, though, and Nelson said they could undermine the credibility of police algorithms.
“The NYPD datasets are infected with deeply rooted biases and racial disparities,” she said. “We are skeptical that such dirty data can ever be cleansed to separate the good from the bad, the tainted from the untainted.”
Nelson also asked the task force to recommend that New York City adopt a uniform definition of automated decision systems, provide public documentation of all algorithms being used by municipal agencies and establish ways to determine if an algorithm is having a disproportionate impact on a specific ethnic group, as well as ways to remedy those effects.
The task force, which is co-chaired by Kelly Jin, the head of the Mayor’s Office of Data Analytics, has until December to issue a report with recommendations, under the law that authorized the panel’s creation. But the task force has been chided for taking nearly a year to hold its first public event and for not sharing details about the closed-door meetings it says it has convened. A group of criminal justice reform and open-government activists sent the task force a brusque letter last August criticizing the lack of public engagement up to that point.
Nelson appeared to flick at the task force’s timeline at the end of her remarks, suggesting the members ask for additional time if needed.
“You have approximately six months to get this right,” she said. “If it takes you longer to do that, I implore you to do that.”
The task force’s next meeting is scheduled May 30.
Correction: A previous version of this article stated the law creating the automated decision system task force was authored by Councilman Peter Koo. The law was authored by Councilman James Vacca.