New toolkit helps governments vet ‘black box’ algorithms for bias
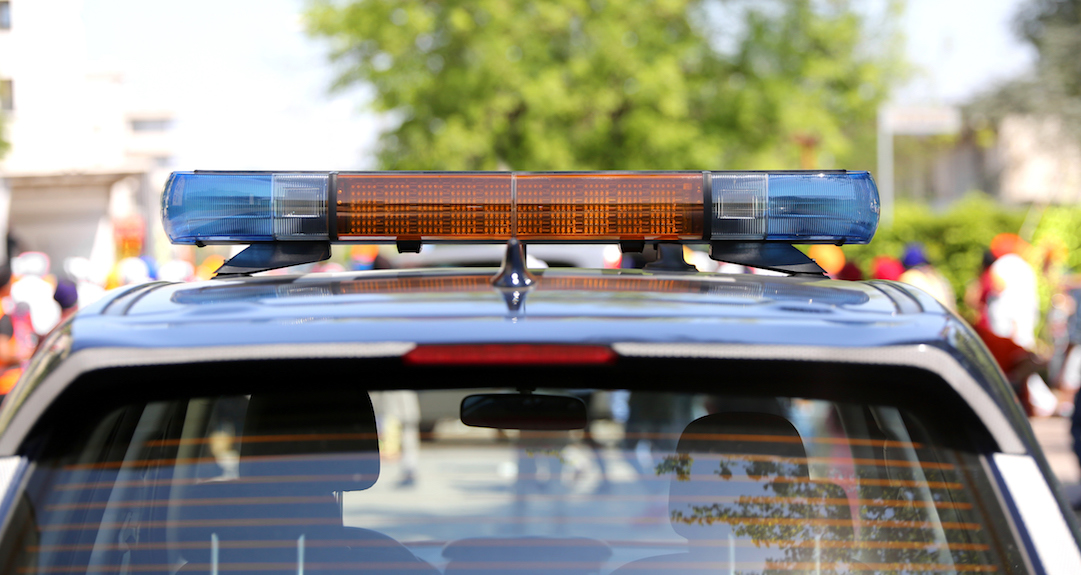
Responding to growing concerns that local governments’ reliance on decision-making software might lead to racially biased policies, a group of researchers released a “toolkit” on Wednesday designed to help agencies use their data in more equitable ways.
The toolkit , built by the Center for Government Excellence at Johns Hopkins University, San Francisco’s data office, the Civic Analytics Network at Harvard University and Data Community DC, was published as a response to reports that algorithms used to make decisions about law enforcement, school enrollment and social services can result in unfair policies when the data behind those decisions are not put into proper context.
“Instead of wringing our hands about ethics and AI, our toolkit puts an approachable and feasible solution in the hands of government practitioners,” Joy Bonaguro, who resigned as San Francisco’s chief data officer on Monday, said in a press release.
The research that went into the toolkit’s development was inspired in part by a 2016 investigation by ProPublica that found that algorithms used by law enforcement agencies in New York and other U.S. cities frequently feed higher incarceration rates among African-American populations. A research paper published by DataSF during the toolkit’s development attributed that effect to what data scientists call “black box” algorithms, processes in which the owners can’t see how decisions are being made and which evaluate individuals without context.
The toolkit contains a “risk-management approach” that urges policymakers to question whether blindly following data results in less efficient delivery of social services or biased policing in minority communities. The first part instructs users to assess an algorithm’s risk by evaluating several factors, including who will be affected by the policy and what kind of impact the government service the algorithm is used for will have. It also evaluates the sources of data being used and the methodology of any third-party vendors that might be involved in the development of an algorithm.
“We background check government employees really sensitively, but we don’t do that same thing for algorithms,” said Andrew Nicklin, the Center for Government Excellence’s director of data practices. “We assume everything will be OK and everyone has the best intentions. But that doesn’t mean they do.”
Nicklin, who previously served in a variety of data and information technology roles for the city and state of New York, said the toolkit isn’t aimed at stopping the use of algorithmic decision making. But it is meant to inject a bit of human control, especially in situations concerning life or death, such as “when you’re talking about someone who might be spending a few extra years in jail,” Nicklin said.
Once a user has gone through the risk assessments, the second half of the toolkit offers 20 potential ways to mitigate algorithmic biases. For instance, with an algorithm used to determine a government service targeting large populations, the toolkit recommends establishing an independent review board or public advisory group. It also suggests tossing out datasets that repeatedly show historic biases. “Stop the controversy before it starts: Do not start a project with data that has the potential to be harmful,” the toolkit reads.
The toolkit is the latest in a string of efforts studying algorithmic bias. In May, New York Mayor Bill de Blasio announced the formation of a 16-person task force that will study the social impact of city agencies’ automated decision-making processes, including those used by the police, education, transportation and social services department. That group is expected to deliver a report by December 2019.
The purpose of all these efforts, Nicklin said, isn’t to question automated decision making entirely, but to subject it to the kind of scrutiny that government services need if they are to be improved.
“We started out with a bunch of assumptions with what we were doing,” Nicklin said. “All data is biased. All algorithms are biased. And all people are biased. If you start from there, you can test some degree of doubt or questioning, as you should.”