Machine learning algorithm triples E. coli prediction rate on Chicago beaches
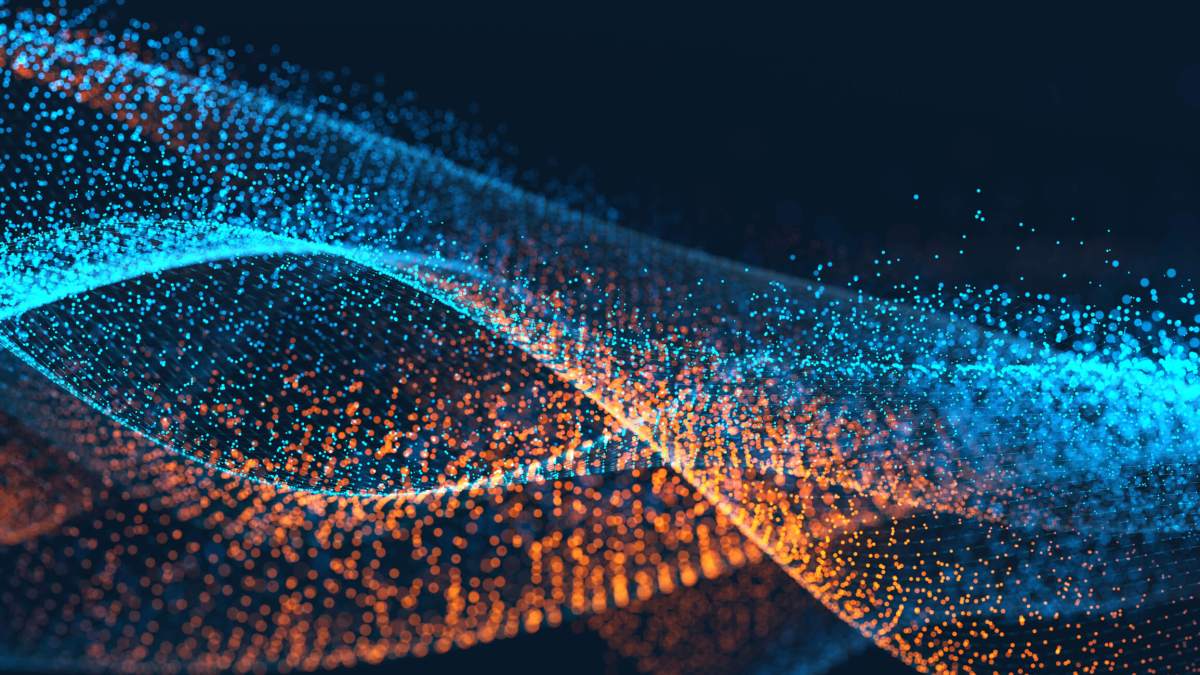
Chicago beachgoers may soon notice that the city is issuing a lot more advisories on unsafe water conditions than it used to. It’s not necessarily that the water quality has gotten worse — it’s that the city’s technology has gotten much better.
Under a test run of a new prediction model fueled by a machine learning algorithm, the City of Chicago’s data division has tripled the accuracy of identifying where potentially deadly E. coli bacteria are contaminating beaches, city Chief Data Officer Tom Schenk told StateScoop. The open-source project, developed in part by local programmers and students who volunteered more than 1,000 hours of work, is giving city officials a more realistic look at water quality in public spaces. It will also save time and money, they said.
After testing, which ran through the beginning of this summer, the city realized it had been vastly under-warning the public of unsafe water conditions. The new model works by interpreting patterns in the results of DNA tests at select beaches along the city’s 26 miles of public Lake Michigan shoreline. The results are then used in combination with analysis of 10 years of historical data to forecast the conditions at untested beaches.
During the testing period this summer, the city continued issuing advisories using the old model. Had the new, more accurate prediction model been used — and not just tested — the city would have issued 69 public advisories instead of just nine, according to the data division.
With more than 60 million annual visitors to Chicago’s 27 beaches, the city’s traditional prediction methods were found to be both inadequate and prohibitively expensive. Traditional testing involves culturing of live E. coli bacteria cells, which can take 18 to 24 hours, while DNA testing can be completed in less than four hours, according to the Chicago Park District. While daily rapid testing is the preferred method for the city because water quality conditions can change rapidly, testing every beach every day is expensive.
That’s where the city’s data division came in. Only half as many beaches need to be tested when using the model powered by the city’s machine learning algorithm — which was written in the open source programming language known as R and is hosted on Github. Just five of the city’s beaches were identified as responsible for more than half of all poor water quality days. By testing those sites and pairing the results with the new prediction model, the city says it has achieved a 12 percent prediction rate, up from four percent under the previous method. By “clustering algorithms,” the city predicts it can achieve accuracy rates that exceed 20 percent.
The recent findings follow an announcement by the parks department earlier this summer that it would begin using DNA testing so it could avoid warning the public about what the water was like yesterday. Bacteria counts exceeding 1,000 “calibrator cell equivalents” — typically caused by feces from dogs, seagulls, or babies, and compounded by runoff caused by heavy rains — are enough to trigger a public advisory.
The city created and tested the new statistical model with the help of volunteers who came from a weekly civic tech meetup called Chi Hack Night, interns from DePaul University’s Masters in Predictive Analytics program, and students from DePaul’s Data Visualization course.