How the NYPD is using machine learning to spot crime patterns
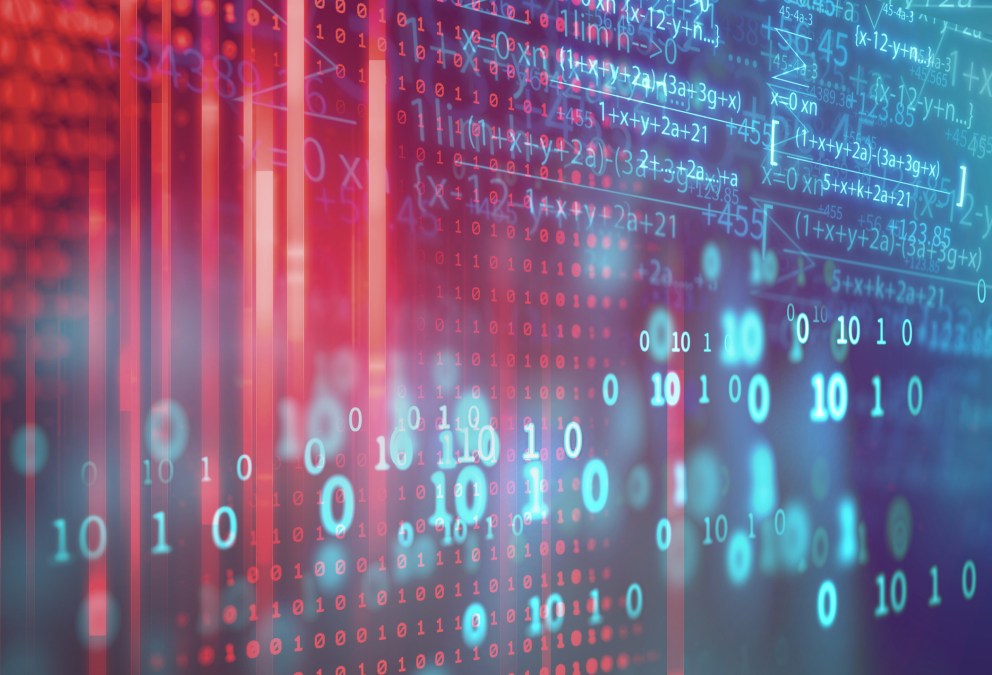
Civilian analysts and officers within the New York City Police Department are using a unique computational tool to spot patterns in crime data that is closing cases.
A collection of machine-learning models, which the department calls Patternizr, was first deployed in December 2016, but the department only revealed the system last month when its developers published a research paper in the Informs Journal on Applied Analytics. Drawing on 10 years of historical data about burglary, robbery and grand larceny, the tool is the first of its kind to be used by law enforcement, the developers wrote.
The NYPD hired 100 civilian analysts in 2017 to use Patternizr. It’s also available to all officers through the department’s Domain Awareness System, a citywide network of sensors, databases, devices, software and other technical infrastructure. Researchers told StateScoop the tool has generated leads on several cases that traditionally would have stretched officers’ memories and traditional evidence-gathering abilities.
Connecting similar crimes into patterns is a crucial part of gathering evidence and eventually closing in on an arrest, said Evan Levine, the NYPD’s assistant commissioner of data analytics and one of Patternizr’s developers. Taken independently, each crime in a string of crimes may not yield enough evidence to identify a perpetrator, but the work of finding patterns is slow and each officer only has a limited amount of working knowledge surrounding an incident, he said.
“The goal here is to alleviate all that kind of busywork you might have to do to find hits on a pattern,” said Alex Chohlas-Wood, a Patternizr researcher and deputy director of the Computational Policy Lab at Stanford University.
The knowledge of individual officers is limited in scope by dint of the NYPD’s organizational structure. The department divides New York into 77 precincts, and a person who commits crimes across precincts, which often have arbitrary boundaries, is often more difficult to catch because individual beat officers are typically focused on a single neighborhood.
There’s also a lot of data to sift through. In 2016 alone, about 13,000 burglaries, 15,000 robberies and 44,000 grand larcenies were reported across the five boroughs.
Levine said that last month, police used Patternizr to spot a pattern of three knife-point robberies around a Bronx subway station. It would have taken police much longer to connect those crimes manually, Levine said.
The software works by an analyst feeding it “seed” case, which is then compared against a database of hundreds of thousands of crime records that Patternizr has already processed. The tool generates a “similarity score” and returns a rank-ordered list and a map. Analysts can read a few details of each complaint before examining the seed complaint and similar complaints in a detailed side-by-side view or filtering results.
Patternizr looks at dozens of attributes for each complaint, including location, date and time of occurrence, weapon used, and suspects’ height and weight. Race and gender are not included in the models and other variables that could give away sensitive information, such as location, are kept “coarse,” according to the journal article. The researchers wrote that “analysis uncovered no evidence that Patternizr recommends any suspect race at a higher rate than exists with random pairing.”
The models also draw data from complaint narratives, suspect statements and other unstructured text, looking for general similarity between the texts and “rare-word matches.”
In another recent case, an analyst seeded the models with the reported theft of a watch from a locker at a hotel gym in Midtown Manhattan in the late afternoon. Patternizr found three other similar complaints of jewelry and watches removed from lockers in fitness facilities in the late afternoon or early evening in the same area. Police identified two suspects through subsequent review of video footage from the facilities. Researchers say the algorithm likely picked up on the times, geographic proximity, type of location (gyms), and keywords like “locker.”
In addition to solving individual crimes, Levine said Patternizr is an additional tool on top of the department’s data analytics that commanding officers can use to allocate resources.
“If there happens to be a pattern of burglaries, that means we’ll put more cops in that area,” Levine said.
The researchers concluded in their paper that the tool has been well received by the department, writing that analysts created more than 90 preliminary patterns per week between January and July 2018. Officers account for about 20 percent of the tool’s usage.
Debra Piehl, an NYPD senior crime analyst, is quoted in the research paper as saying that “Patternizr dramatically improves efficiency compared to traditional methods, and it still allows the analysts that work for me to apply their own thinking and analysis. The science doesn’t overwhelm the art.”
NYPD plans to expand use of the tool to include petty larceny, Levine said, but there are no plans to use it for violent crimes like murder or homicide, because those crimes already have a lot of resources dedicated to them.
Though Patternizr does not include race or gender data and the NYPD’s tests show no racial bias, the tool is still being criticized as potentially biased. Gartner analyst Darin Stewart told SearchBusiness Analytics that the historical data the models use could be biased and that would be enough to make the models’ outcomes biased. Additionally, the American Civil Liberties Union is unsatisfied with internal testing and is asking for an independent audit of the technology.
Levine and Chohlas-Wood said Patternizr is not only a time-saver for police, but a potential means of averting the flawed memories and naked analyses of human officers and analysts.
“These tools, when used properly, encourage precision policing approaches instead of widespread, heavy-handed enforcement techniques and enable investigators to focus on the art of policing instead of rote and uninteresting work,” the report concluded.