Maryland beefs up data analytics tools to weed out tax fraud
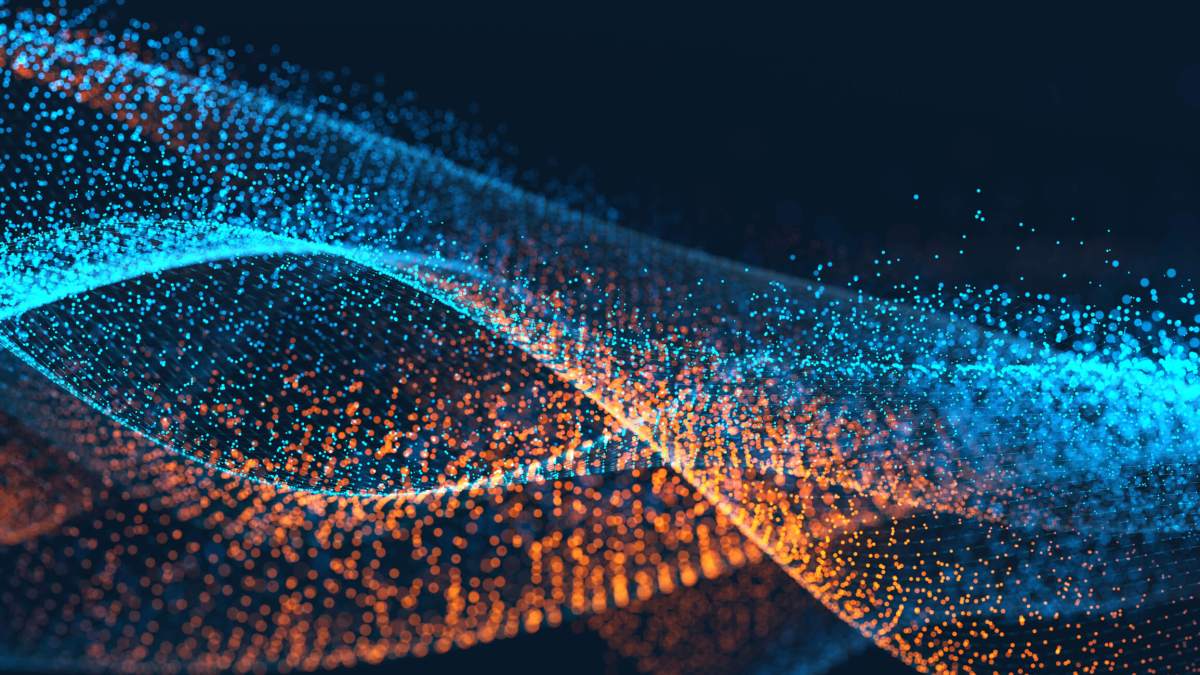
Maryland is changing the way it uses data analytics to fight tax fraud, increasingly relying on predictive models to identify questionable returns and save time while working to filter out fraud.
Though the Comptroller of the Treasury’s office is still reviewing tax returns filed for the past year, Andrew Schaufele — the director of the agency’s Bureau of Revenue Estimates — told StateScoop that the office’s new set of algorithms is now identifying fraudulent returns at “about a 65 to 70 percent” clip. That’s a sizable jump from the group’s 55 percent success rate with its model last year, and lightyears ahead of the 10 percent mark the office managed when it first started experimenting with data analytics several years ago, Schaufele noted.
“At this point in the year, we’ve seen a stark increase in accuracy,” Schaufele said. “And that’s huge, because one of our primary directives here is not only to reduce fraud but to get taxpayers their money as quickly as possible. Most people count on those tax returns. … It’s the most effective poverty-fighting weapon that exists within tax policy.”
Indeed, Schaufele described the new reliance on data analysis as part of a broader push to save time for the office’s “Questionable Return Detection Team.” As people submit their tax documents, the office runs that data through what Schaufele terms a “decision tree” model, which analyzes the returns by comparing them with the state’s existing data on its citizens to find inconsistencies.
Based on the results that tool spits out, the fraud detection team can then prioritize which returns deserve more in-depth review.
“Their work has completely changed because of this model,” Schaufele said.
Schaufele noted that, even though the office has been using a “data warehouse” and working with the data analytics firm Teradata for the last six years, this increasing reliance on the results of the algorithms is a relatively recent shift.
“At the time, we had 15 to 20 different metrics that we used to flag these records for review, things as basic as what is your withholding relative to your total income,” Schaufele said. “Some of those were effective in general, but we were flagging about 110,000 returns each year and we have a staff of about 20.”
That workload was overwhelming for the office’s fraud analysts. Though the system was helping the office stop 11,000 fraudulent returns each year, the early algorithms were casting far too wide a net, Schaufele said.
Accordingly, Schaufele began to work with Teradata’s researchers to study the data they’d collected on the fraudulent returns to start refining the office’s model.
“As we began to learn about the fraud, we found consistencies amongst the fraud and developed a regression,” Schaufele said. “We were scoring returns, finding a probability that it was fraud, and we set a threshold in our system, and started flagging returns.”
[Read more: Georgia, North Carolina take aim at tax fraud with new app]
But Schaufele noted that many in the office were still hesitant to fully embrace the new scoring model, and they continued to flag far more returns than they needed to out of caution.
“Change did take some time,” Schaufele said. “As with any government, there’s extraordinary bureaucracy, and there is entrenchment in existing practice. Like most state governments, most of our employees have been here for 25 to 30 years, so they know one way of doing things.”
However, by tax time last year, Schaufele was able to develop the new decision tree model, and persuaded the office to solely use the tool to send returns to the review team. He said that led to a rousing success, as the office flagged just 33,000 returns for analysis, hitting at a 55 percent accuracy rate and stopping $24 million in fraud in the process. Those sorts of results even earned the project a StateScoop 50 award, which honors IT innovations from the past year.
“We ended up with a sizable return with about a third of the manual effort, and we were quite happy with that,” Schaufele said.
They didn’t stop there. The office spent the past year adding new features, including new “profiles for taxpayers,” which Schaufele said the office uses to help uncover identity theft.
“Let’s say you normally file in Maryland, you have a direct deposit, we know your bank for several years has been this,” Schaufele said. “We’ve added a component where we’re checking the likelihood that this is you.”
He said they’ve also begun clustering returns by “ZIP codes and tax preparers,” helping them to identify patterns of fraud, and even move to try and prevent it.
“We found very high instances of fraud at certain tax preparation facilities, so we began to investigate that and we’ve now stopped accepting returns from 60 tax preparers that were sending a preponderance of questionable returns,” Schaufele said. “This is something no other state has done, the IRS has never done this, so this is kind of uncharted territory here.”
Schaufele noted the office has also used the model to reprioritize the order in which analysts evaluate returns.
“If you have a high fraud likelihood score, we work you last,” Schaufele said. “We’re going to get to people that we think are more on the line, so if it’s a false positive, we can get it out to them.”
Yet for all these advances, Schaufele said his office can make the model even more robust in time for next year’s tax season. In particular, he sees organized fraud rings as the biggest threat on the horizon for the state, and he hopes to refine the office’s model to weed out these mass-produced fraud attempts going forward.
“I think that’s the next step, building mechanisms to make sure we’re rooting out this organized fraud and trying to stay ahead of them,” Schaufele said. “We’ve just got to continue to adapt.”
Contact the reporter at alex.koma@statescoop.com, and follow him on Twitter @AlexKomaSNG.