Has generative AI made our best privacy principles obsolete?
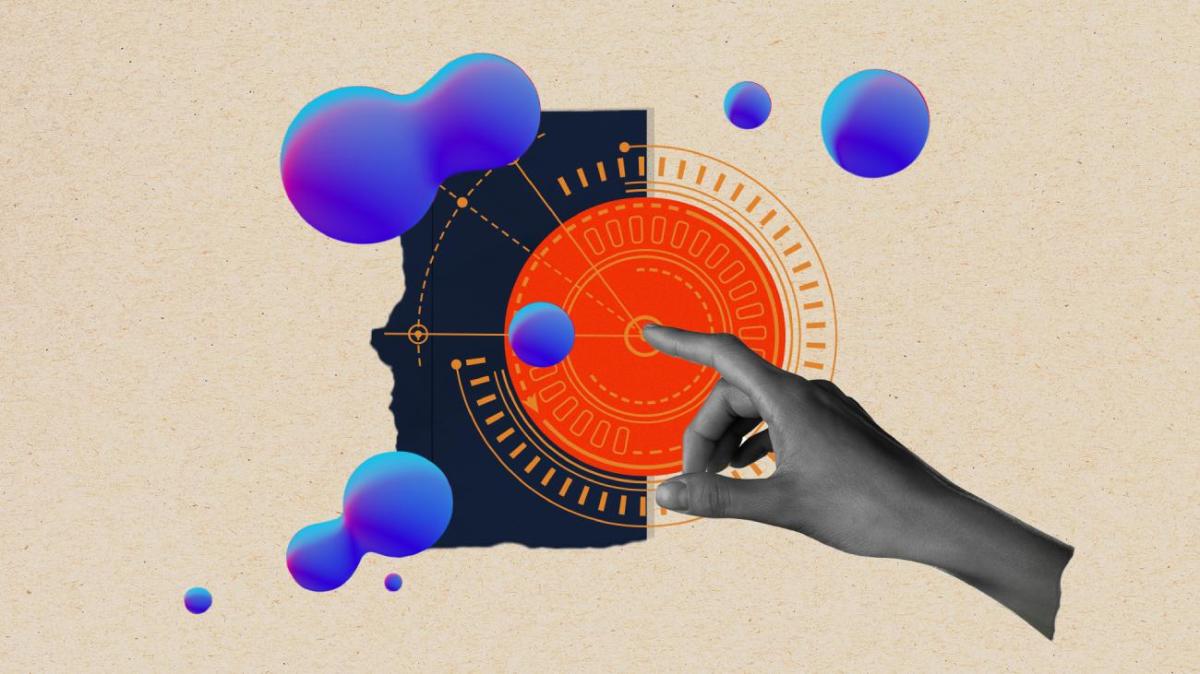
The Organization for Economic Cooperation and Development’s privacy principles were, in many ways, a masterpiece. Written in 1980, they set out clear and specific guidelines that gave rise to the first comprehensive privacy laws. Core concepts such as accountability, transparency, and data security all spring from this source: if these ideas seem obvious today, it’s a reflection of how deeply ingrained the OECD’s guidelines are in the way that both consumers and businesses think about data privacy.
Look at the OECD’s principles on AI use, which were updated last year, and you’ll see a very different story playing out. Compared with the precise language and clear principles in the 1980 privacy guidelines, the AI principles are almost comically hesitant. They offer only the broadest of goals — encouraging the creation of “trustworthy AI” that doesn’t actively break the law, for instance — with little or no guidance on how to enact them, or even judge whether they’ve been achieved.
Its recommendations are hedged with the repeated proviso that they only apply when “appropriate to the context and consistent with the state of the art” — a shrugging acknowledgement that AI is so new, and evolving so fast, that any specific principles would be outdated before the ink was dry.
The contrast between these two sets of guidelines couldn’t be clearer. When the privacy principles were adopted, the OECD could essentially ignore technical challenges and simply articulate how data should or shouldn’t be treated. In the AI era, though, tech is evolving so fast that any concrete guidelines risk becoming immediately obsolete. Like other regulatory groups, the OECD decided its only option was to articulate broad values, rather than give clear guidance on how they should be implemented.
Like it or not, we live in the AI era, and that’s complicating data privacy just as much as it’s complicating efforts to regulate AI itself. As we think about the future of privacy in an AI world, it’s important to ask: are the OECD’s privacy principles still relevant? Or are they becoming obsolete — a regulatory weapon from a more civilized age, before AI made a mockery of attempts to pass clear and prescriptive recommendations?
The OECD’s privacy principles are grounded in the idea that every single bit of personal data is unique, important and deserving of individual protection. From my bank information to my facial biometrics, the idea goes, my information is mine and needs to be handled with the utmost caution and respect.
For AI innovators, however, the world looks very different. Instead of worrying about individual droplets of data, AI concerns itself with the swirling tides and currents of the entire ocean. My data may not matter much at all, on an individual level: What matters is data in aggregate and the patterns and signals that can be coaxed out of vast datasets.
That simple distinction lays waste to the OECD’s privacy principles.
The collection limitation principle calls for restraint in the collection of personal data, and for data to only be collected with the subject’s knowledge and consent, while the data quality principle says we should only collect the data needed to achieve our goals. AI depends, however, on indiscriminately ingesting vast amounts of data, including colossal quantities of public data scraped without notifying anyone.
The purpose specification principle says we should disclose our goals up front, and the use limitation principle says personal data shouldn’t be used for purposes other than those specified during collection. But AI depends on collecting data first and then figuring out what’s possible to do with it — so unless we’re willing to accept “doing AI stuff” as a legitimate purpose, both these principles go out the window.
The security safeguards principle says personal data should be protected against loss or unauthorized access, and the individual participation principle says individuals have the right to know what’s being done with their data and to have their data amended or deleted. But AI models embed data in their own algorithms in ways that can’t simply be disclosed or deleted.
The openness principle says developers should work openly and transparently, and the accountability principle says a data controller should be accountable for enacting all the foregoing principles. In an era of black-box algorithms, where not even the developer really knows what’s going on under the hood, both these principles are virtually impossible to implement in any meaningful way.
Regulating AI using the OECD’s existing privacy principles will be about as effective as using traffic laws to halt a supernova. Case in point: The French Data Protection Authority got tied up in knots recently by trying to argue that data minimization doesn’t preclude training AI models on big datasets — but does still require developers to avoid feeding “unnecessary” personal data into AI systems. That brings us straight back to the core question of how, exactly, AI developers can know in advance whether data was necessary or not.
Because the privacy industry is on a collision course with a technological and economic juggernaut, clinging too hard to the OECD’s principles could wind up doing more harm than good. If privacy advocates commit to a privacy framework that’s fundamentally incompatible with the AI revolution, the entire framework could wind up getting left behind. If we force people to choose between privacy and AI, we could wind up with AI and no privacy.
That would be a disaster, because the the values underpinning the OECD’s principles remain incredibly important. Precisely because AI radically complicates everything, and makes the OECD’s privacy principles virtually impossible to enforce as written, the underlying ideals of transparency and dignity and fairness and agency are needed more than ever. (Data privacy is dead — long live data privacy!)
I don’t have a satisfying solution to offer — like you, I’m just one more drop in the ocean. But I believe this is the conversation our industry needs to be having if we want to avoid sacrificing data privacy on the altar of AI innovation. Pretending that old approaches to data privacy are enough will backfire fast: If we want privacy to endure in the AI era, we need to get serious and start rethinking the foundational ideas on which modern privacy infrastructure is built.
This is a submission from a contributor and does not necessarily reflect the opinions of Scoop News Group.
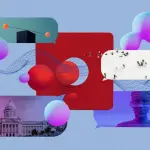
This story was featured in StateScoop Special Report: Artificial Intelligence 2024